This year’s panel at the the 2017 PRG Hardware Symposium included leading Artificial Intelligence and Hardware Product experts from a broad range of industries:
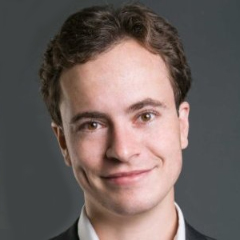
Alastair Trueger, founder of Creative Ventures (moderator)
Luke Tang, General Manager, TechCode AI Accelerator
Radhika Dirks, Partner, Xlabs.ai
Mark Jacobstein, Chief User Engagement Officer at Guardant Health
Greg Reichow, General Partner, Eclipse Ventures and former VP of Manufacturing at Tesla Motors
Revolution or Evolution? Some claim AI software is waiting for the hardware to catch up. Others claim the the opposite. Moderator Alastair Trueger asked our panelists.
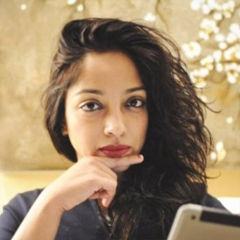
According to Radhika Dirks, Partner at Xlabs.ai, when artificial intelligence applications move beyond mimicking human intelligence to going beyond human intelligence, you start beginning to think about designing hardware differently. Radhika took DNA as an example, looking at how “it computes.” She explained that nature provides an elegant solution where storage, coding and processing happens in the same hardware. Nonetheless, she pondered, “is the reduction of a human neuron to 1’s, 0’s and binary output really the right way to bottle it? How would hardware look like when we start developing for the future?” According to Radhika, AI applications that are solving for the the past are not waiting for hardware to catch up. On the other hard, AI application that are trying to solve for future problems are absolutely waiting for hardware to catch up.
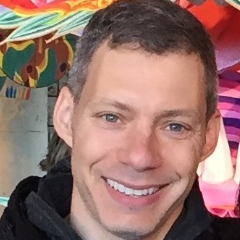
For Mark Jacobstein, Chief User Engagement Officer at Guardant Health, those in the medical fields are definitely waiting for hardware advances in AI. Chronic disease management and other areas that can be managed with continuous monitoring are areas waiting for advances. Pieces of hardware exist to monitor the glucose level of diabetic patient continuously. Similarly, a pulse oximeter can be used to measure the blood and oxygen levels of someone who suffers from COPD. But according Mark, the current hardware versions are not tiny, not sexy, and not easy to wear, and advances are at least 5-7 years out. Machine learning will be critical in diagnosis and crisis aversion. The continuous monitoring piece is a sensor problem- it’s hardware.
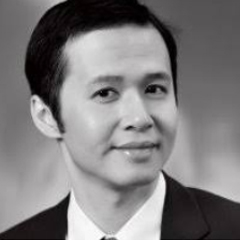
From Luke Tang’s perspective, it’s all relative: you can argue both ways. Luke, the General Manager at TechCode AI Accelerator, explains that for existing hardware, they are waiting for new algorithms (software) that can train with smaller data sets or maybe do unsupervised learning. For existing algorithms, we’re waiting for better hardware. For example, for deep reasoning, we need to improve the hardware for better access to memory and to store complex data sets and models.
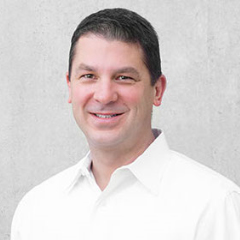
While not completely disagreeing with the other panelists, Greg Reichow, General Partner, Eclipse Ventures and former VP of Manufacturing at Tesla Motors, offers a counterpoint. According to Greg, there’s another subtle part to this discussion that comes from looking at the problems being solved now and how effectively we are using the hardware. He concludes that it’s pretty awful, that is, we are not really effectively using the CPU power that we have today. Even when we try to optimize, for example with, dedicated machine learning rigs, the actual percentage effective use of the theoretical processing power is pretty low.
Greg thinks that there is a lot of of thinking to do about how the software and the architecture can better utilize the hardware. The thinking goes to what kinds of algorithms are we trying to develop and how they actually function, and what they imply for the hardware and software that will solve those problems. As Greg notes, there are lots of problems, lots of variables, and how we solve for them, whether it be quantum computing, the next hardware platform, etc.– different algorithms will be an important part of making hardware.